Understanding Enterprise Data Governance: Part 2
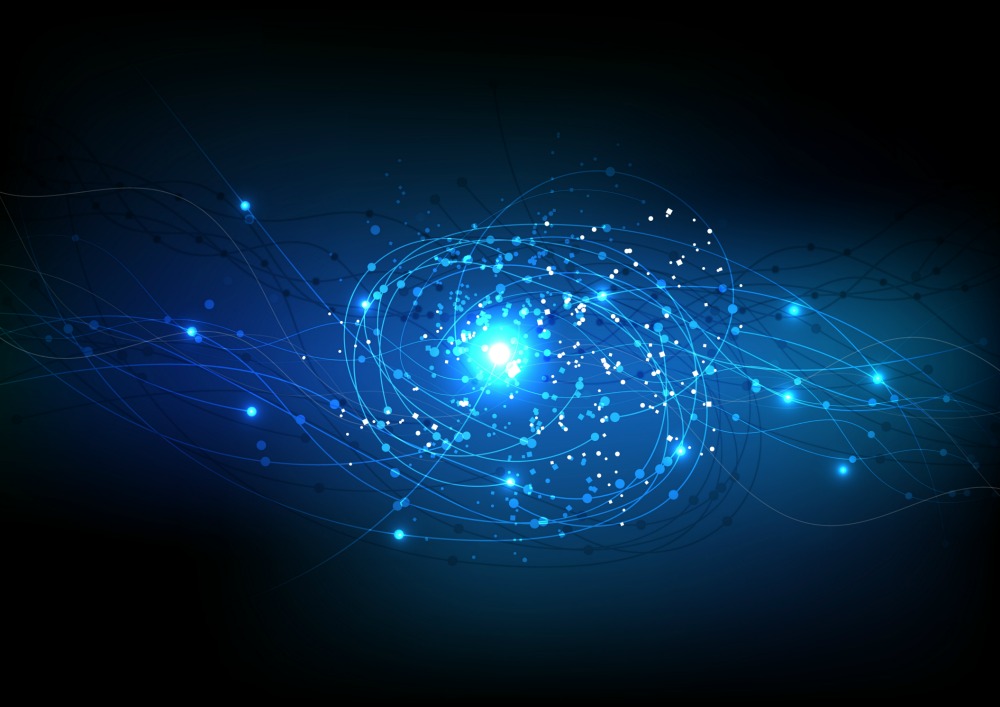
This is the second blog post in a series exploring Enterprise Data Governance. In the first post, we briefly defined transaction data, metadata, master data, reference data, and dimensional data. That discussion primarily focused on transactional data and metadata, and can be found here. In this post, we will further explore reference data and its role in data governance solutions.
As we move beyond transaction data and metadata, and into the realms of master and reference data, most academics and analysts tend to focus on solutions and methodologies rather than attempting to clearly differentiate between the types of data that need to be governed. Not only does this introduce a solution bias, but it also leads to a tendency to lump these data categories together in master/slave relationships and leave it at that. For example, reference data is commonly classified as a subset of master data, and dimensional data as a subset of reference data.
Technically, there is nothing inaccurate about these assertions, but it would be a mistake to think that a single solution can fully address all of them without first gaining an understanding of the different challenges involved in governing these various types of data. Only then can we accurately assess the solutions and technologies that are best suited to the task. For this purpose, we will treat master data, reference data, and dimensional data as separate, distinct categories from a governance perspective.
Reference data is the easiest of the three types to understand. It is made up of various lists and code sets that are used to classify and organize data. Country codes, industry codes, status codes, account types, and employee types are among the many examples of reference data. Reference data sets can vary wildly in size and complexity. For example, there might only be a dozen or so valid account status codes, whereas there may be over a thousand valid industry codes. Code sets related to product SKUs, financial instruments, and the like can be much larger, ranging into the hundreds of thousands or even millions of records in rare cases.
While the concept of reference data is easy to grasp, there can also be significant complexities that need to be addressed. Some reference data sets are standardized by regulatory, or governing bodies, such as the International Standards Organization, which maintains standardized lists of country codes among other things.
Another example is the US Census Bureau, which maintains the North American Industry Classification System (NAICS). It is common for companies to require internally managed alternate code sets as well. For example, an COTS solution may include US territories in an internal State table, requiring this alternate list to be cross-referenced to standardized state and territory code sets for regulatory purposes.
Other reference data sets need to be controlled directly by the enterprise since they relate to how business is conducted. Sales territories, lines of business, and departments are common examples. As mentioned previously with the State table example, this can also include the configurations of code sets within applications, such as employee types and account status, when custom business processes need to be accommodated.
From a governance perspective, mastering reference data goes beyond maintaining traditional lookup tables. The ability to maintain well-documented business and technical definitions of code set values, including data versioning and audit history, are essential. Functionalities for maintaining and validating mappings between related code sets are also of vital importance.
Keep watch for the third part of this blog series, Understanding Enterprise Data Governance!
Leave a Reply